This insight was featured in the March 5th, 2025 edition of the AI Catalyst Pulse.
This is the second in a four-part series examining the critical strategic dilemmas healthcare executives must navigate in 2025. Read part one here.
Every AI strategy must balance control against innovation. This creates healthcare's second fundamental AI dilemma in 2025: Do you maintain tight central oversight of AI deployment, or do you empower your frontline teams with the freedom to experiment and innovate?
As with all dilemmas in this series, “central oversight vs. frontline freedom” is to some extent a false binary; every health system needs some combination of both approaches. However, our conversations with AI Catalyst members consistently reveal that budgets, attention spans, and implementation capacity are limited — forcing tough choices about strategic emphasis.
The case for central governance: One unified AI strategy
The basic argument for centralizing AI governance is simple: The most reliable way to create a consistent, safe, and compliant AI strategy is to put one body in charge of AI decisions.
Most health systems seem to be moving in this direction. When we surveyed 2024 AI Bootcamp attendees, 52% told us their systems have “a formalized, system-wide AI steering committee.” Among the benefits of this approach:
An empowered AI steering committee can make coordinated, strategic bets. According to McKinsey, 75% of organizations list digital transformation as a high priority but admit they haven't sufficiently planned or resourced these initiatives. Without central coordination, departments risk pursuing duplicative AI projects even as strategic priorities go unfunded.
A central “veto point” can save you from dangerous AI blunders. To give one scary example: Texas's attorney general recently alleged that an AI vendor "made deceptive claims about the accuracy of its healthcare AI products, putting the public interest at risk." If you have a well-informed governance committee reviewing every new AI tool, you’re more likely to catch these risks before they impact patients.
A well-structured oversight body can ensure that important voices are represented in every AI decision. For instance, HCA's recent contract with National Nurses United provides nurses formal representation on the system's AI Governance Committee — guaranteeing that frontline perspectives shape all AI adoption decisions.
Central governance structures make it easier to comply with fast-changing legal requirements. Lawmakers are considering an ever-expanding list of requirements for AI in healthcare, such as standardized disclosures of how AI tools are trained. A central governance committee makes it easier to meet these demands.
An organization-wide oversight body can identify cross-departmental benefits that individual teams might miss. When departments pursue AI in isolation, they naturally focus on their own pain points — and may miss opportunities to make an enterprise-wide impact. For example, while clinical documentation AI tools are often championed by physicians for burnout reduction, they can also deliver big revenue cycle benefits through improved coding.
So what might a centralized AI governance committee look like in practice? Consider UW Health’s Clinical AI & Predictive Analytics Committee, which includes representatives from bioethics, law, biostatistics, data science, clinical operations, and DEI. For implementation, the organization created specialized "algorithm sub-committees" that work directly with requesters to oversee specific use cases — maintaining central control while engaging domain expertise.
The case for frontline freedom: Innovation needs room to breathe
Despite these benefits, there are important reasons not to centralize your AI governance. If you want to ensure your AI applications solve real-world clinical and operational problems, you need to give your teams the freedom to experiment and iterate.
A more frontline-driven approach addresses several key challenges:
When central approval processes become too cumbersome, frontline workers may create (potentially dangerous) workarounds. When your official AI processes require months of committee reviews, clinicians and administrators will find unauthorized shortcuts — creating far greater risk than controlled departmental experimentation.
The people closest to the work can best understand which AI tools will make a real difference.
A nurse manager or service line director knows exactly where documentation burdens are heaviest or where communication breakdowns occur.
Many central governance frameworks demand resources health systems simply don't have.
One AI executive we spoke with put it bluntly: "In the world I work in, I do not have the resources to implement the level of governance coming out of [current frameworks]."
Providence illustrates what a more decentralized approach to AI can look like in action. Their governance model establishes basic checkpoints while empowering frontline "idea champions" to drive AI projects forward. These champions identify use cases, complete basic templates, and partner with technical guides throughout development — treating governance as guidance rather than a permission-granting bureaucracy.
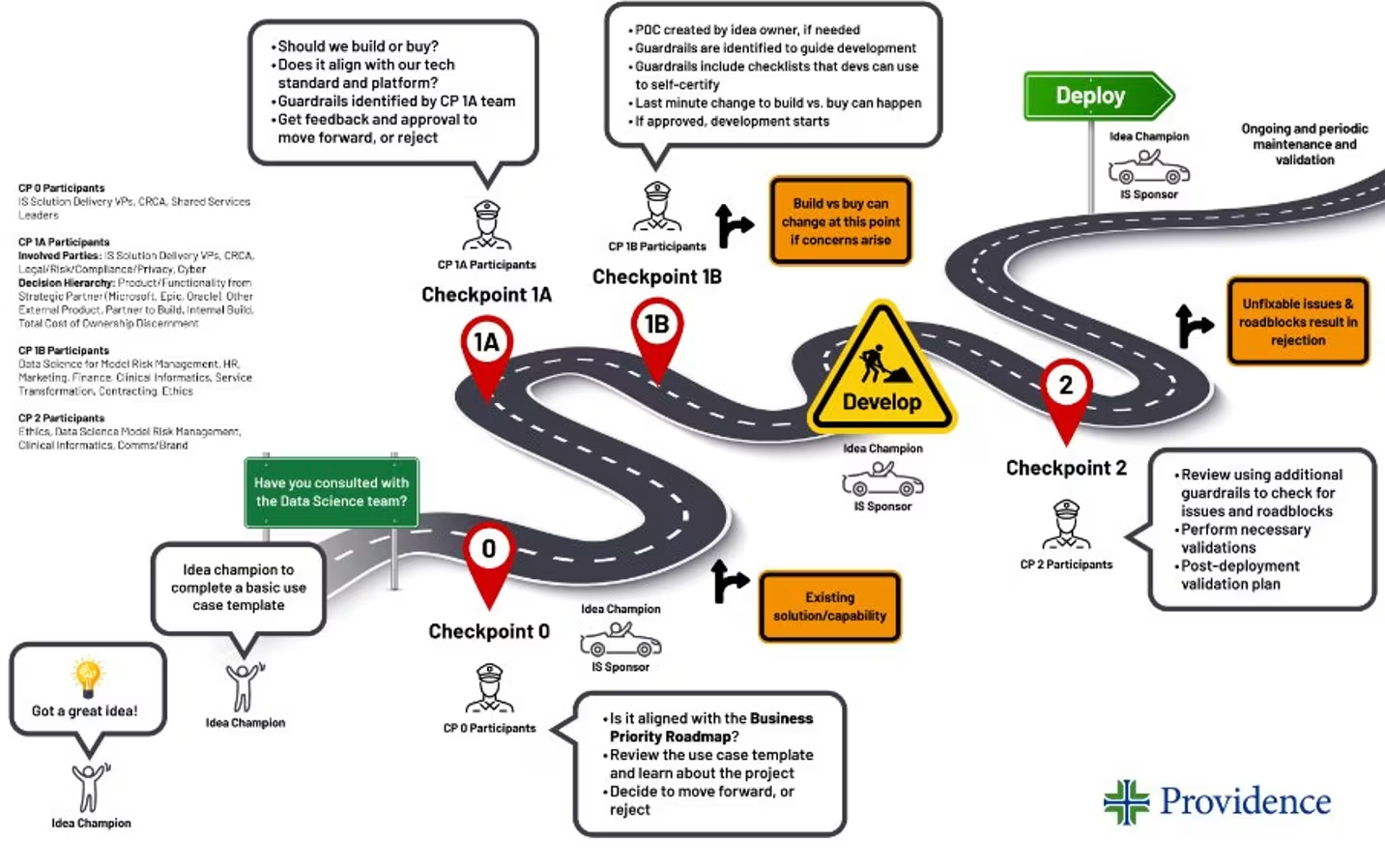
Which should you choose: Central oversight or frontline freedom?
Every health system needs both central guidance and frontline innovation, but you need to choose an emphasis that fits your organization.
Our guidance: Consider prioritizing central oversight if you have established governance structures you can build upon (such as a Digital Health Taskforce), or if your organization is taking its first steps into AI and needs to carefully manage early risks. This approach makes particular sense for organizations focused on just a few big, focused AI bets. If you’re pursuing a centralized approach:
Identify strong functional representatives from clinical, IT, revenue cycle, and other key areas — and expect meaningful time commitment from these individuals.
Create a risk-tiered review process where high-risk clinical applications receive full committee scrutiny, while lower-risk tools follow a more streamlined path.
Consider establishing a controlled "sandbox" environment for low-risk frontline experiments to prevent shadow AI use while still enabling innovation.
On the other hand, consider prioritizing frontline freedom if your staff demonstrates strong AI literacy and enthusiasm, or if your organization has a culture that traditionally rewards responsible experimentation. This approach works best when you trust your divisional leaders to understand and enforce appropriate guidelines. In that case:
Create standardized, practical tools for frontline leaders — such as vendor assessment questionnaires and risk scoring templates — that help them make good decisions independently.
Invest heavily in AI literacy education to ensure department-level decision-makers understand both AI's potential and its limitations.
Implement regular auditing processes to identify both successful use cases worth scaling and potential risks requiring intervention.
So where do top health system leaders come down on the “centralized governance” vs. “frontline freedom” dilemma? When we posed the question at The Health Management Academy’s recent Trustee Summit, fully 72% of health system CEOs and board members preferred a centralized strategy.
Yet a few health systems are finding ways to split the difference. Mass General Brigham, for instance, combines a system-level AI Governance Committee with subcommittees integrating frontline technical and clinical considerations. Adventist Health demonstrates another hybrid approach: Clinical AI applications must undergo rigorous, centralized scrutiny, while operational AI tools follow a more streamlined path.
Ultimately, AI oversight requires bidirectional communication. Department leaders need to understand system-level risks, but just as importantly, system leaders must pursue strategies that capture benefits within and beyond individual departments.
Questions to consider:
If you conducted a candid audit of your governance approach, what gaps would you find between your formal structures and how AI is actually being implemented?
Where have you seen frontline teams using AI successfully without central oversight? What lessons could you draw from these examples?
How effectively does your current governance approach identify AI use cases with benefits that span multiple departments?
Does your AI governance approach reflect your organization's overall culture and decision-making style, or are you imposing a clashing governance style?